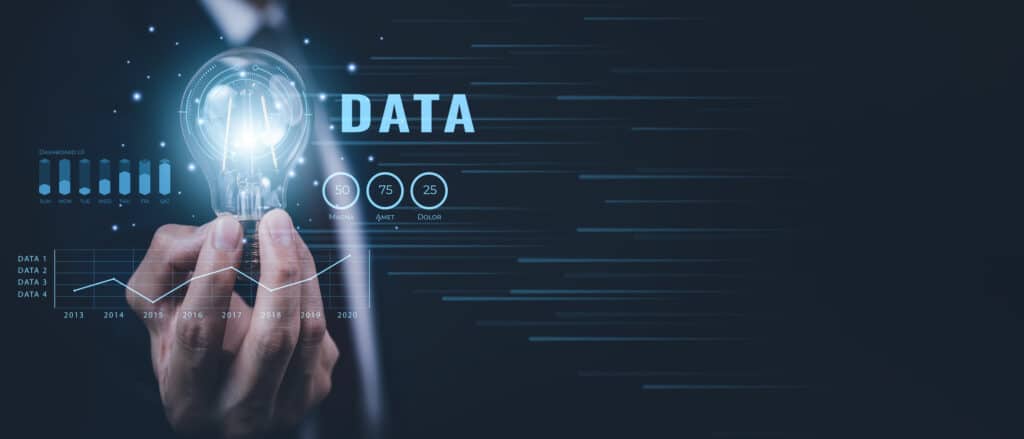
Welcome to the first part of our three-part blog series on DataOps 101. In today's rapidly evolving digital landscape, organizations are collecting and generating vast amounts of data. However, merely amassing data is not enough to gain insights and drive value. To harness the power of data effectively, businesses need efficient and streamlined processes. That's where DataOps comes into play. In this blog post, we will delve into the fundamentals of DataOps, its significance, and how it enables organizations to thrive in the data-driven era.
Understanding DataOps:
DataOps is an emerging methodology that combines principles from agile development, DevOps, and data management to optimize the entire data lifecycle. It emphasizes collaboration, automation, and continuous improvement in data-related processes (Just like IDS’ People, Processes and Technologies). Just as DevOps revolutionized software development by integrating development and operations teams, DataOps aims to bridge the gap between data engineering, data science, and business operations.
Understanding The Need for DataOps:
Traditional approaches to data management often involve siloed departments, fragmented workflows, and a lack of collaboration. These inefficiencies can lead to delays, errors, and missed opportunities. DataOps, with its holistic approach, addresses these challenges and provides a framework for streamlined data operations.
Agility and Speed:
DataOps promotes agility and speed by fostering collaboration among different teams involved in the data lifecycle. By breaking down silos and facilitating cross-functional communication, DataOps enables faster development and deployment of data-driven solutions. It encourages iterative processes and empowers teams to respond quickly to changing business needs.
Data Quality and Reliability:
DataOps emphasizes the importance of data quality and reliability. By implementing automated testing and monitoring, organizations can identify and rectify data issues in real-time. This ensures that the data used for analysis and decision-making is accurate, consistent, and reliable, leading to more confident and informed decision-making processes.
Scalability and Efficiency:
With the exponential growth of data, organizations need scalable and efficient systems to handle the ever-increasing volumes of information. DataOps promotes automation and orchestration, allowing organizations to manage data pipelines and workflows more effectively. By automating repetitive tasks and leveraging cloud technologies, DataOps enables scalability while reducing operational costs.
Collaboration and Alignment:
DataOps breaks down barriers between different teams involved in the data lifecycle, including data engineers, data scientists, business analysts, and operations teams. It encourages collaboration and alignment, fostering a shared understanding of data-related goals and objectives. By facilitating communication and knowledge sharing, DataOps promotes a data-driven culture within the organization.
DataOps is a transformative methodology that addresses the challenges organizations face in managing and leveraging their data effectively. By combining agile principles, automation, and cross-functional collaboration, DataOps enables organizations to unlock the full potential of their data assets. In the next part of this series, we will explore the key components and best practices of DataOps implementation. Stay tuned to learn how you can embrace DataOps to drive innovation and success in your organization.
References:
Chen, M., Mao, S., & Liu, Y. (2018). Big data: a survey. Mobile networks and applications, 19(2), 171-209.
Marz, N., & Warren, J. (2015). Big data: principles and best practices of scalable real-time data systems.
Manning Publications Co.
Trewin, Simon (2022). The DataOps Revolution- Delivering the Data-Driven Enterprise.